Hi, my name is Mark. I’ve been a member of ACE for almost 9 years. There’s been three things on my To-Do list gnawing at my psyche for some time:
- Learn about Raspberry Pi microprocessors through Internet of Things (IoT) applications.
- Get hands-on experience with Artificial Intelligence.
- Learn the popular Python programming language.
Why these? Because computers are getting smaller while getting more powerful; Artificial Intelligence (AI) is running on ever smaller computers; and Python is a versatile, beginner-friendly language that’s well-documented and used for both Raspberry Pi (RPi) and AI projects.
I’ve been working in computer vision, a field of AI, for several years in both business development and business operations capacities. While I don’t have a technical background, I strive to understand how the engineering of products & services of my employers works in order to facilitate communication with clients. Throughout my career I’ve asked a lot of engineers a lot of naive questions because I’m curious about how the underlying technologies come together on a fundamental level. I owe a big thanks to those engineers for their patience with me! It was time for me to learn it by doing it on my own.
Computer Vision gives machines the ability to see the world as humans do – Using methods for acquiring, processing, analyzing, and understanding digital images or spatial information.
In starting on my learning journey I began a routine of studying at our ACE Makerspace coworking space every week to be around other makers. This helped me maintain focus after the a pandemic induced a work-from-home lifestyle that left me inhibited by a serious brain fog.
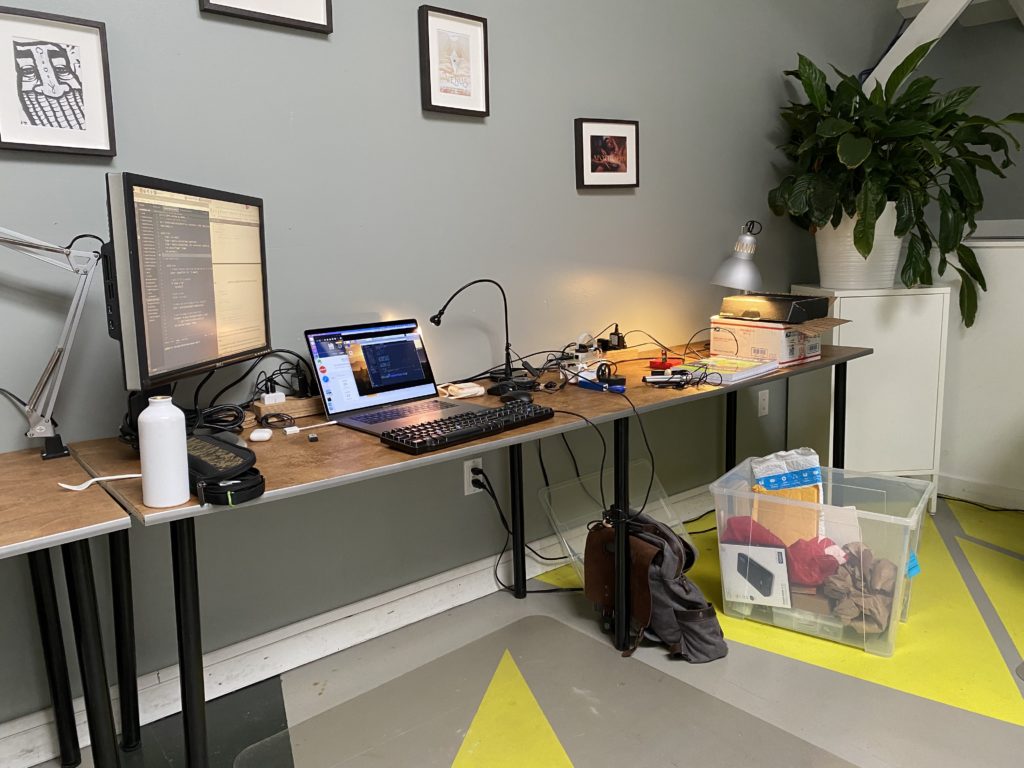
OpenCV (Open Source Computer Vision Library) is a cross-platform library of programming functions mainly aimed at real-time computer vision. AMONG MANY COMPONENTS It includes a machine learning library as a set of functions for statistical classification, regression, and clustering of data.
Fun Fact: Our ACE Makerspace Edgy Cam Photobooth seen at many ACE events uses an ‘Edge Detection’ technique also from the OpenCV Library.
A self-paced Intro to Python course came first. Then came a course on OpenCV which taught the fundamentals of image processing. Later still came tutorials on how to train a computer to recognize objects, and even faces, from a series of images.
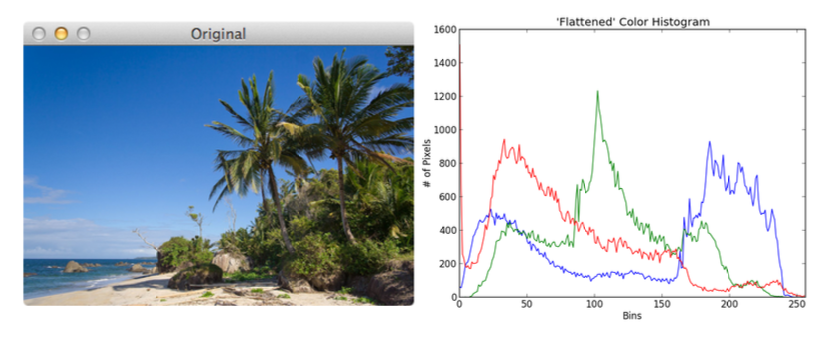
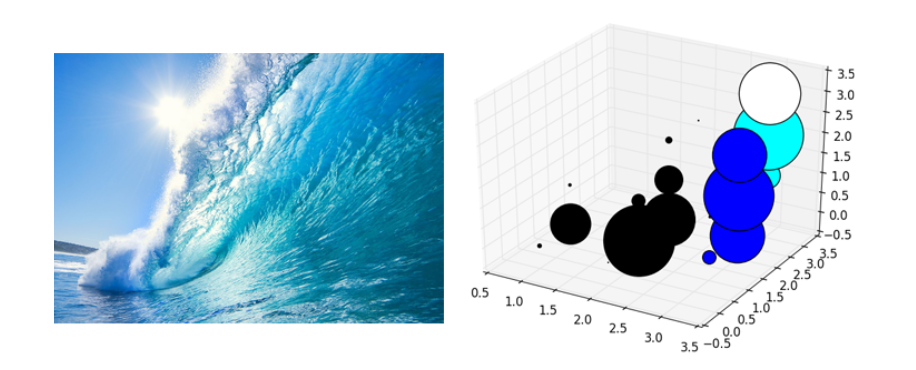
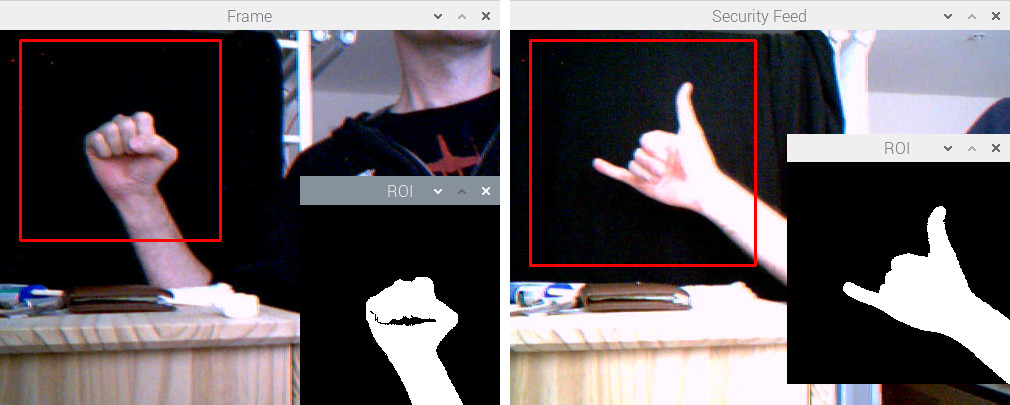
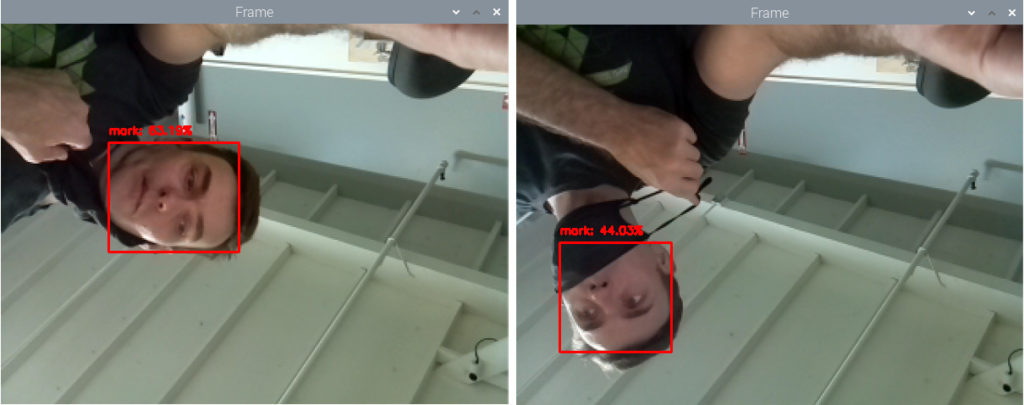
Eventually, I moved onto more complex projects, including programming an autonomous mini robot car that responds to commands based on what the AI algorithm infers from an attached camera’s video feed – This was real-time computer vision! There were many starter robot car kits to choose from. Some are for educational purposes, others come pre-assembled with a chassis, motor controllers, sensors, and even software. Surely, this was the best path for me to get straight into the software and image processing. But the pandemic had bogged down supply chains, and it seemed that any product with a microchip was on backorder for months.
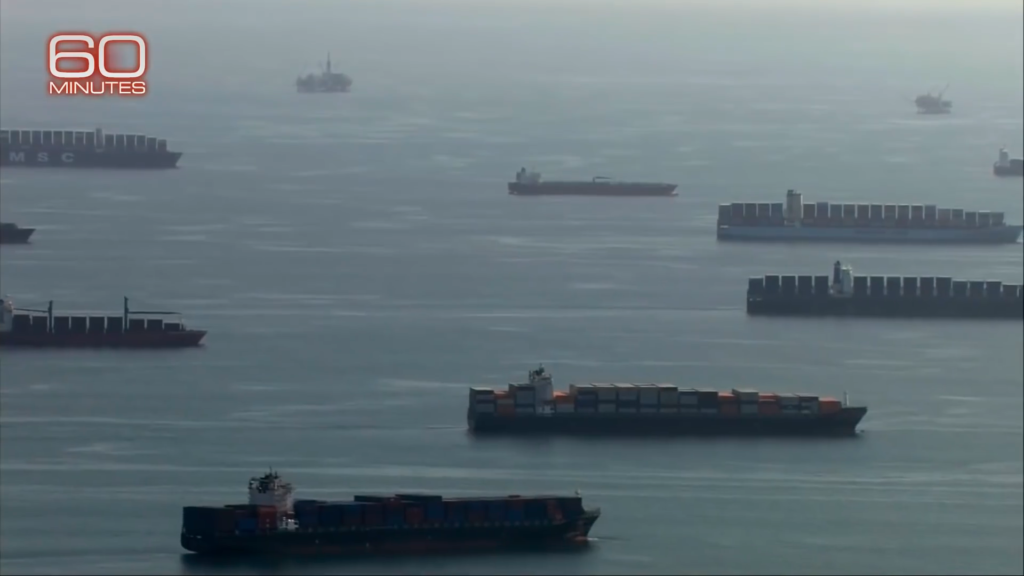
I couldn’t find a starter robot car kit for sale online that shipped within 60 days and I wasn’t willing to wait that long. And I didn’t want to skip this tutorial because it was a great exercise combining the RPi, AI, and Python programming triad. ACE Makerspace facilities came to the rescue again with the electronics stations and 3D printers which opened up my options.
I learned a few things working at computer vision hardware companies: Sometimes compromises are made in hardware due to availability of components; Sometimes compromises are made in the software due to the lack of time. One thing was for sure, I had to decide on an alternative hardware solution because hardware supply was the limiting factor. On the other hand, software was rather easy to modify to work with various motor controllers.
So after some research I decided on making my own robot car kit using the JetBot reference design. The JetBot is an open-source robot based on the Nvidia Jetson Nano, another single board computer more powerful than the RPi. Would this design work with the RPi? I ordered the components and shifted focus to 3D printing the car chassis and mounts while waiting for components from Adafruit and Amazon to arrive. ACE has (2) Prusa 3D printers on which I could run print jobs in parallel;
When the parts arrived I switched over to assembling and soldering (and in my case, de-soldering and re-soldering) the electronic components using ACE’s electronics stations equipped with many of the hand tools, soldering materials, and miscellaneous electrical components. When fully assembled, swapping in the Raspberry Pi for the Jetson Nano computer was simple and it booted up and operated as described on the JetBot site.
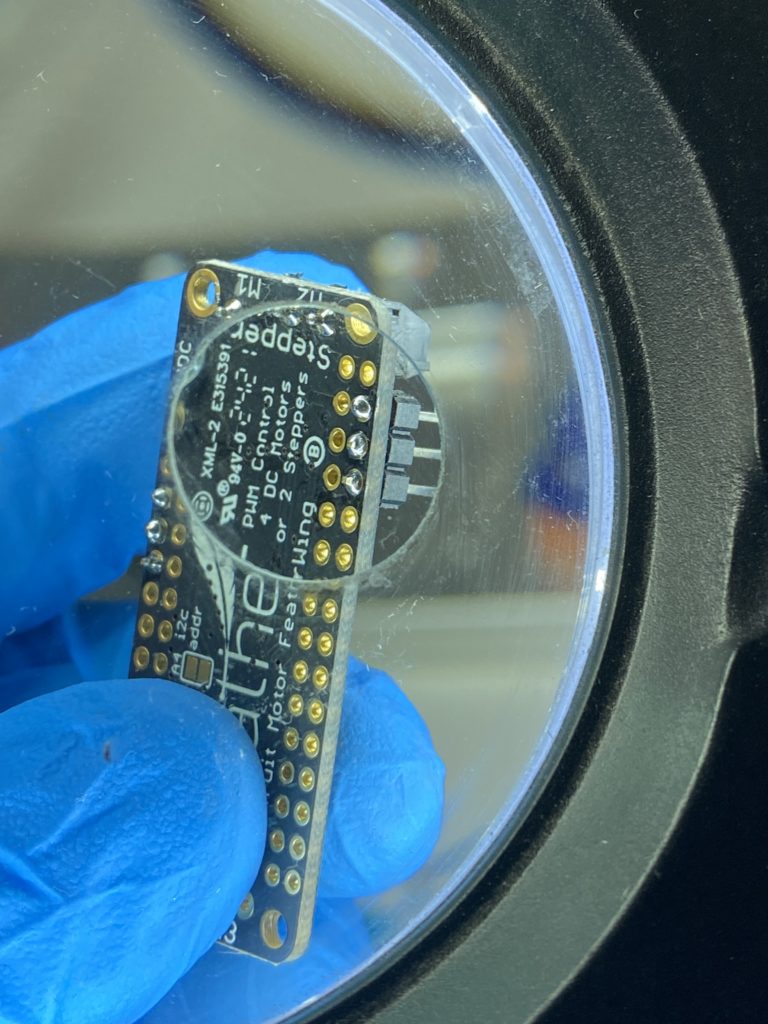
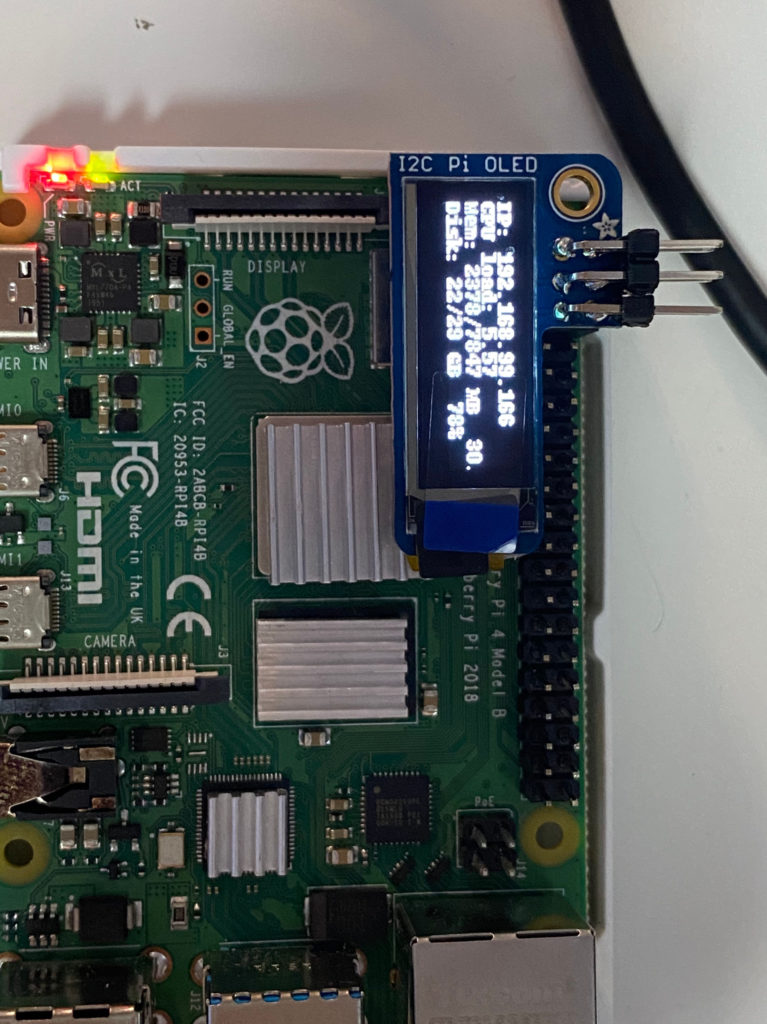
The autonomous robot car starts by roaming around at a constant speed in a single direction. The Raspberry Pi drives the motor controls, operates the attached camera, and marshals the camera frames to the attached blue coprocessor, an Intel Neural Compute Stick (NCS), plugged into and powered by the Raspberry Pi USB 3.0 port. It’s this NCS that is “looking” for a type of object in each camera frame. The NCS is a coprocessor dedicated to the application-specific task of object detection using a pre-installed program called a MobileNet SSD – pre-trained to recognize a list of common objects. I chose the object type ‘bottle’.
“MobileNet” because they are designed for resource constrained devices such as your smartphone. “SSD” stands for “Single-shot Detector” because object localization and classification are done in a single forward pass of the neural network. In general, single-stage detectors tend to be less accurate than two-stage detectors, but are significantly faster.
The Neural Compute Stick’s processor is designed to perform the AI inference – accurately detecting and correctly classifying a ‘bottle’ in the camera frame. The NCS localizes the bottle within the camera frame and determines the bounding box coordinates of where in the frame the object is located. The NCS then sends these coordinates to the RPi; The RPi reads these coordinates, determines the center of the bounding box and whether that single center point is to the Left or Right of the center of the RPi’s camera frame.
Knowing this, the RPi will steer the robot accordingly by sending separate commands to the motor controller that drives the two wheels:
- If that Center Point is Left of Center, then the motor controller will slow down the left wheel and speed up the right wheel;
- If that Center Point is Right of Center, then the motor controller will slow down the right wheel and speed up the left wheel;
Keeping the bottle in the center of the frame, the RPi drives the car towards the bottle. In the lower-right corner of the video below is a picture-in-picture video from the camera on the Raspberry Pi. A ‘bottle’ is correctly detected and classified in the camera frames. The software [mostly] steers the car towards the bottle.
Older USB Accelerators, such as the NCS (v1), can be slow and cause latency in the reaction time of the computer. So there’s a latency in executing motor control commands. (Not a big deal for a tabletop autonomous mini-car application, but it is a BIG deal for autonomous cars being tested in the real world on the roads today.) On the other hand, this would be difficult to perform on the RPi alone, without a coprocessor, because the Intel NCS is engineered to perform the application-specific number-crunching more efficiently and while using less power than the CPU on the Raspberry Pi.
Finally, I couldn’t help but think that there was some irony in this supply chain dilemma that I had experienced while waiting for electronics to help me learn about robots; Because maybe employing more robots in factories will be how U.S. manufacturers improve resilience of supply chains if these companies decide to “onshore” or “reshore” production back onto home turf. Just my opinion.
Since finishing this robot mini-car I’ve moved on to learn other AI frameworks and even training AI with data in the cloud. My next challenge might be to add a 3D depth sensor to the robot car and map the room in 3D while applying AI to the depth data. A little while back I picked up a used Neato XV-11 robot vacuum from an ACE member, and I might start exploring that device for its LIDAR sensor instead.
Let me know if you’re interested in learning about AI or microprocessors, or if you’re working on similar projects. Until then, I’ll see you around ACE!
Mark Piszczor
LinkedIn